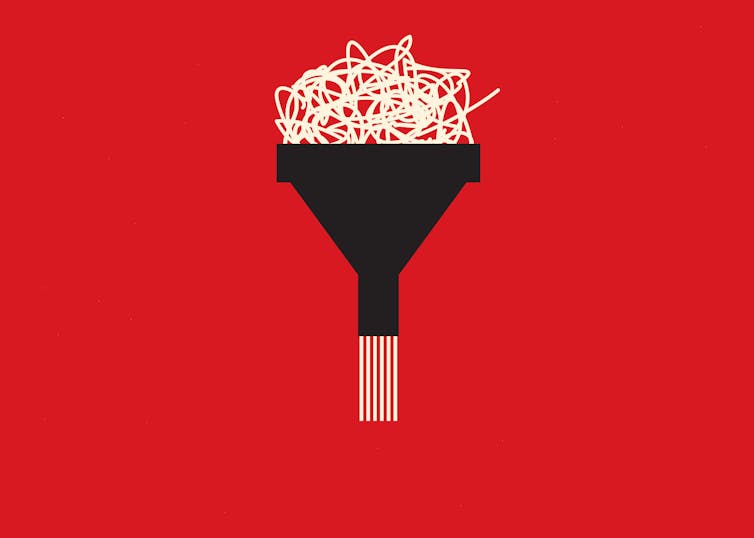
kislev/iStock via Getty Images Plus
David Markowitz, Michigan State University
Artificial intelligence-generated summaries of scientific papers make complex information more understandable for the public compared with human-written summaries, according to my recent paper published in PNAS Nexus. AI-generated summaries not only improved public comprehension of science but also enhanced how people perceived scientists.
I used a popular large language model, GPT-4 by OpenAI, to create simple summaries of scientific papers; this kind of text is often called a significance statement. The AI-generated summaries used simpler language – they were easier to read according to a readability index and used more common words, like “job” instead of “occupation” – than summaries written by the researchers who had done the work.
In one experiment, I found that readers of the AI-generated statements had a better understanding of the science, and they provided more detailed, accurate summaries of the content than readers of the human-written statements.
I also investigated what effects the simpler summaries might have on people’s perceptions of the scientists who performed the research. In this experiment, participants rated the scientists whose work was described in the simpler texts as more credible and trustworthy than the scientists whose work was described in the more complex texts.
In both experiments, participants did not know who wrote each summary. The simpler texts were always AI-generated, and the complex texts were always human-generated. When I asked participants who they believed wrote each summary, they ironically thought the more complex ones were written by AI and simpler ones were written by humans.
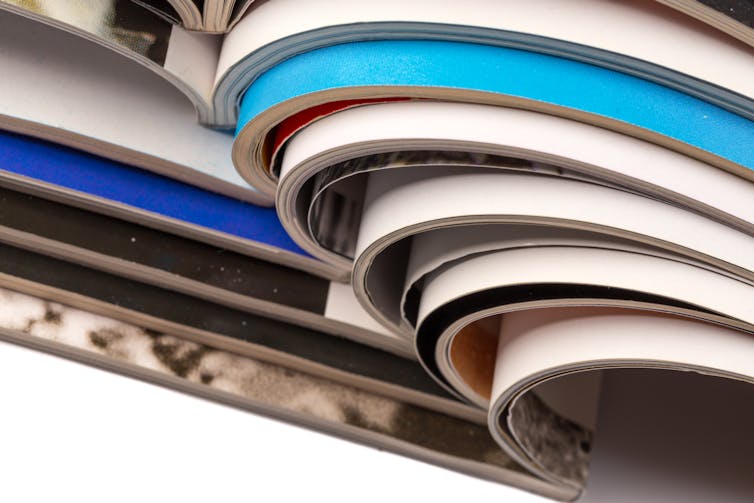
R.Tsubin/Moment via Getty Images
Why it matters
Have you ever read about a scientific discovery and felt like it was written in a foreign language? If you’re like most Americans, new scientific information is probably hard to understand – especially if you try to tackle a science article in a research journal.
In an era where scientific literacy is crucial for informed decision-making, the abilities to communicate and grasp complex ideas are more important than ever. Trust in science has been declining for years, and one contributing factor may be the challenge of understanding scientific jargon.
This research points to a potential solution: using AI to simplify science communication. By making scientific content more approachable, this work demonstrates that AI-generated summaries may help to restore trust in scientists and, in turn, encourage greater public engagement with scientific issues. The question of trust is particularly important, as people often rely on science in their daily lives, from eating habits to medical choices.
What still isn’t known
As AI continues to evolve, its role in science communication may expand, especially if using generative AI becomes more commonplace or sanctioned by journals. Indeed, the academic publishing field is still establishing norms regarding the use of AI. By simplifying scientific writing, AI could contribute to more engagement with complex issues.
While the benefits of AI-generated science communication are perhaps clear, ethical considerations must also be considered. There is some risk that relying on AI to simplify scientific content may remove nuance, potentially leading to misunderstandings or oversimplifications. There’s always the chance of errors, too, if no one pays close attention.
Additionally, transparency is critical. Readers should be informed when AI is used to generate summaries to avoid potential biases.
Simple science descriptions are preferable to and more beneficial than complex ones, and AI tools can help. But scientists could also achieve the same goals by working harder to minimize jargon and communicate clearly – no AI necessary.
David Markowitz, Associate Professor of Communication, Michigan State University
This article is republished from The Conversation under a Creative Commons license. Read the original article.