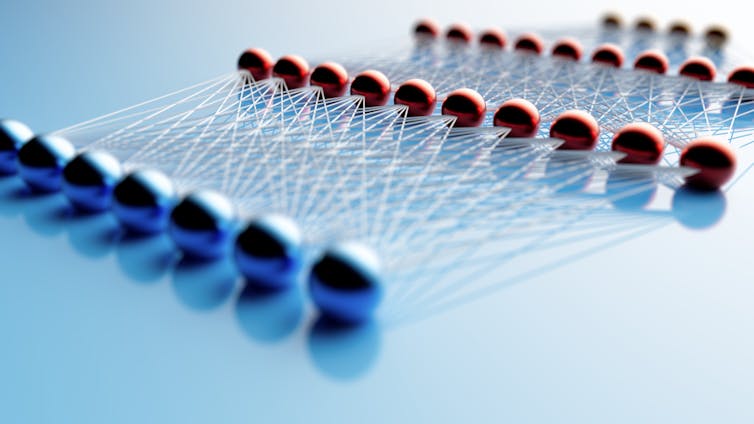
Thom Leach/Science Photo Library via Getty Images
Ambuj Tewari, University of Michigan
If your jaw dropped as you watched the latest AI-generated video, your bank balance was saved from criminals by a fraud detection system, or your day was made a little easier because you were able to dictate a text message on the run, you have many scientists, mathematicians and engineers to thank.
But two names stand out for foundational contributions to the deep learning technology that makes those experiences possible: Princeton University physicist John Hopfield and University of Toronto computer scientist Geoffrey Hinton.
The two researchers were awarded the Nobel Prize in physics on Oct. 8, 2024, for their pioneering work in the field of artificial neural networks. Though artificial neural networks are modeled on biological neural networks, both researchers’ work drew on statistical physics, hence the prize in physics.
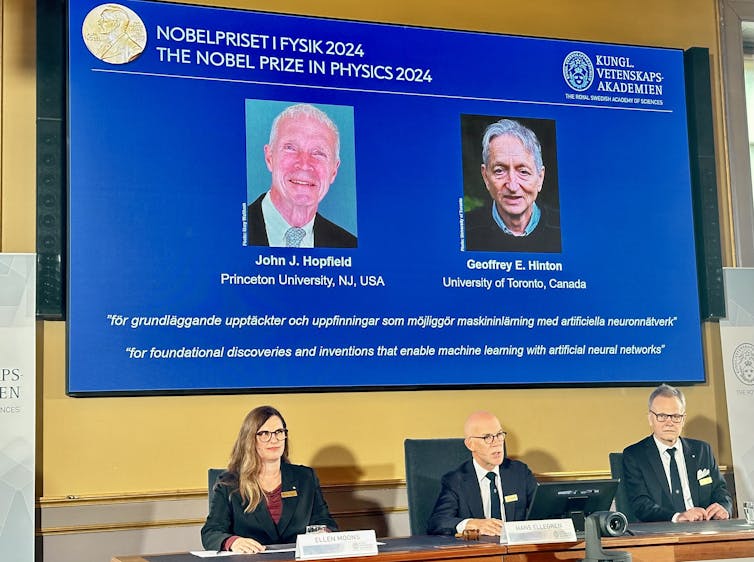
Atila Altuntas/Anadolu via Getty Images
How a neuron computes
Artificial neural networks owe their origins to studies of biological neurons in living brains. In 1943, neurophysiologist Warren McCulloch and logician Walter Pitts proposed a simple model of how a neuron works. In the McCulloch-Pitts model, a neuron is connected to its neighboring neurons and can receive signals from them. It can then combine those signals to send signals to other neurons.
But there is a twist: It can weigh signals coming from different neighbors differently. Imagine that you are trying to decide whether to buy a new bestselling phone. You talk to your friends and ask them for their recommendations. A simple strategy is to collect all friend recommendations and decide to go along with whatever the majority says. For example, you ask three friends, Alice, Bob and Charlie, and they say yay, yay and nay, respectively. This leads you to a decision to buy the phone because you have two yays and one nay.
However, you might trust some friends more because they have in-depth knowledge of technical gadgets. So you might decide to give more weight to their recommendations. For example, if Charlie is very knowledgeable, you might count his nay three times and now your decision is to not buy the phone – two yays and three nays. If you’re unfortunate to have a friend whom you completely distrust in technical gadget matters, you might even assign them a negative weight. So their yay counts as a nay and their nay counts as a yay.
Once you’ve made your own decision about whether the new phone is a good choice, other friends can ask you for your recommendation. Similarly, in artificial and biological neural networks, neurons can aggregate signals from their neighbors and send a signal to other neurons. This capability leads to a key distinction: Is there a cycle in the network? For example, if I ask Alice, Bob and Charlie today, and tomorrow Alice asks me for my recommendation, then there is a cycle: from Alice to me, and from me back to Alice.
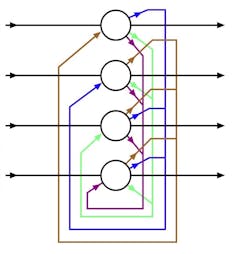
Zawersh/Wikimedia, CC BY-SA
If the connections between neurons do not have a cycle, then computer scientists call it a feedforward neural network. The neurons in a feedforward network can be arranged in layers. The first layer consists of the inputs. The second layer receives its signals from the first layer and so on. The last layer represents the outputs of the network.
However, if there is a cycle in the network, computer scientists call it a recurrent neural network, and the arrangements of neurons can be more complicated than in feedforward neural networks.
Hopfield network
The initial inspiration for artificial neural networks came from biology, but soon other fields started to shape their development. These included logic, mathematics and physics. The physicist John Hopfield used ideas from physics to study a particular type of recurrent neural network, now called the Hopfield network. In particular, he studied their dynamics: What happens to the network over time?
Such dynamics are also important when information spreads through social networks. Everyone’s aware of memes going viral and echo chambers forming in online social networks. These are all collective phenomena that ultimately arise from simple information exchanges between people in the network.
Hopfield was a pioneer in using models from physics, especially those developed to study magnetism, to understand the dynamics of recurrent neural networks. He also showed that their dynamics can give such neural networks a form of memory.
Boltzmann machines and backpropagation
During the 1980s, Geoffrey Hinton, computational neurobiologist Terrence Sejnowski and others extended Hopfield’s ideas to create a new class of models called Boltzmann machines, named for the 19th-century physicist Ludwig Boltzmann. As the name implies, the design of these models is rooted in the statistical physics pioneered by Boltzmann. Unlike Hopfield networks that could store patterns and correct errors in patterns – like a spellchecker does – Boltzmann machines could generate new patterns, thereby planting the seeds of the modern generative AI revolution.
Hinton was also part of another breakthrough that happened in the 1980s: backpropagation. If you want artificial neural networks to do interesting tasks, you have to somehow choose the right weights for the connections between artificial neurons. Backpropagation is a key algorithm that makes it possible to select weights based on the performance of the network on a training dataset. However, it remained challenging to train artificial neural networks with many layers.
In the 2000s, Hinton and his co-workers cleverly used Boltzmann machines to train multilayer networks by first pretraining the network layer by layer and then using another fine-tuning algorithm on top of the pretrained network to further adjust the weights. Multilayered networks were rechristened deep networks, and the deep learning revolution had begun.
AI pays it back to physics
The Nobel Prize in physics shows how ideas from physics contributed to the rise of deep learning. Now deep learning has begun to pay its due back to physics by enabling accurate and fast simulations of systems ranging from molecules and materials all the way to the entire Earth’s climate.
By awarding the Nobel Prize in physics to Hopfield and Hinton, the prize committee has signaled its hope in humanity’s potential to use these advances to promote human well-being and to build a sustainable world.
Ambuj Tewari, Professor of Statistics, University of Michigan
This article is republished from The Conversation under a Creative Commons license. Read the original article.