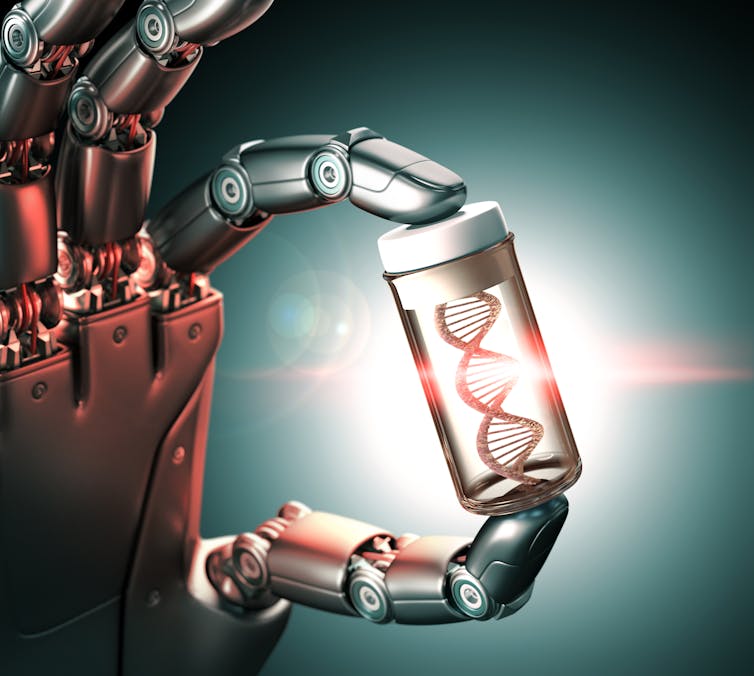
KTSFotos/Moment via Getty Images
Marc Zimmer, Connecticut College
During her chemistry Nobel Prize lecture in 2018, Frances Arnold said, “Today we can for all practical purposes read, write and edit any sequence of DNA, but we cannot compose it.” That isn’t true anymore.
Since then, science and technology have progressed so much that artificial intelligence has learned to compose DNA, and with genetically modified bacteria, scientists are on their way to designing and making bespoke proteins.
The goal is that with AI’s designing talents and gene editing’s engineering abilities, scientists can modify bacteria to act as mini factories producing new proteins that can reduce greenhouse gases, digest plastics or act as species-specific pesticides.
As a chemistry professor and computational chemist who studies molecular science and environmental chemistry, I believe that advances in AI and gene editing make this a realistic possibility.
Gene sequencing – reading life’s recipes
All living things contain genetic materials – DNA and RNA – that provide the hereditary information needed to replicate themselves and make proteins. Proteins constitute 75% of human dry weight. They make up muscles, enzymes, hormones, blood, hair and cartilage. Understanding proteins means understanding much of biology. The order of nucleotide bases in DNA, or RNA in some viruses, encodes this information, and genomic sequencing technologies identify the order of these bases.
The Human Genome Project was an international effort that sequenced the entire human genome from 1990 to 2003. Thanks to rapidly improving technologies, it took seven years to sequence the first 1% of the genome and another seven years for the remaining 99%. By 2003, scientists had the complete sequence of the 3 billion nucleotide base pairs coding for 20,000 to 25,000 genes in the human genome.
However, understanding the functions of most proteins and correcting their malfunctions remained a challenge.
AI learns proteins
Each protein’s shape is critical to its function and is determined by the sequence of its amino acids, which is in turn determined by the gene’s nucleotide sequence. Misfolded proteins have the wrong shape and can cause illnesses such as neurodegenerative diseases, cystic fibrosis and Type 2 diabetes. Understanding these diseases and developing treatments requires knowledge of protein shapes.
Before 2016, the only way to determine the shape of a protein was through X-ray crystallography, a laboratory technique that uses the diffraction of X-rays by single crystals to determine the precise arrangement of atoms and molecules in three dimensions in a molecule. At that time, the structure of about 200,000 proteins had been determined by crystallography, costing billions of dollars.
AlphaFold, a machine learning program, used these crystal structures as a training set to determine the shape of the proteins from their nucleotide sequences. And in less than a year, the program calculated the protein structures of all 214 million genes that have been sequenced and published. The protein structures AlphaFold determined have all been released in a freely available database.
To effectively address noninfectious diseases and design new drugs, scientists need more detailed knowledge of how proteins, especially enzymes, bind small molecules. Enzymes are protein catalysts that enable and regulate biochemical reactions.
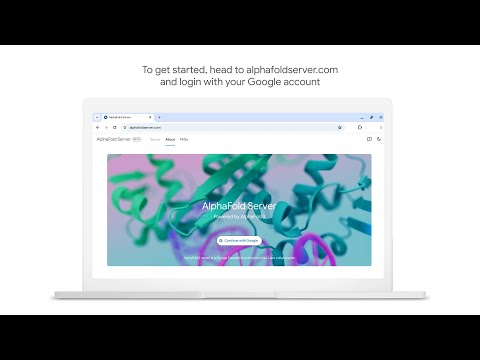
AlphaFold3, released May 8, 2024, can predict protein shapes and the locations where small molecules can bind to these proteins. In rational drug design, drugs are designed to bind proteins involved in a pathway related to the disease being treated. The small molecule drugs bind to the protein binding site and modulate its activity, thereby influencing the disease path. By being able to predict protein binding sites, AlphaFold3 will enhance researchers’ drug development capabilities.
AI + CRISPR = composing new proteins
Around 2015, the development of CRISPR technology revolutionized gene editing. CRISPR can be used to find a specific part of a gene, change or delete it, make the cell express more or less of its gene product, or even add an utterly foreign gene in its place.
In 2020, Jennifer Doudna and Emmanuelle Charpentier received the Nobel Prize in chemistry “for the development of a method (CRISPR) for genome editing.” With CRISPR, gene editing, which once took years and was species specific, costly and laborious, can now be done in days and for a fraction of the cost.
AI and genetic engineering are advancing rapidly. What was once complicated and expensive is now routine. Looking ahead, the dream is of bespoke proteins designed and produced by a combination of machine learning and CRISPR-modified bacteria. AI would design the proteins, and bacteria altered using CRISPR would produce the proteins. Enzymes produced this way could potentially breathe in carbon dioxide and methane while exhaling organic feedstocks, or break down plastics into substitutes for concrete.
I believe that these ambitions are not unrealistic, given that genetically modified organisms already account for 2% of the U.S. economy in agriculture and pharmaceuticals.
Two groups have made functioning enzymes from scratch that were designed by differing AI systems. David Baker’s Institute for Protein Design at the University of Washington devised a new deep-learning-based protein design strategy it named “family-wide hallucination,” which they used to make a unique light-emitting enzyme. Meanwhile, biotech startup Profluent, has used an AI trained from the sum of all CRISPR-Cas knowledge to design new functioning genome editors.
If AI can learn to make new CRISPR systems as well as bioluminescent enzymes that work and have never been seen on Earth, there is hope that pairing CRISPR with AI can be used to design other new bespoke enzymes. Although the CRISPR-AI combination is still in its infancy, once it matures it is likely to be highly beneficial and could even help the world tackle climate change.
It’s important to remember, however, that the more powerful a technology is, the greater the risks it poses. Also, humans have not been very successful at engineering nature due to the complexity and interconnectedness of natural systems, which often leads to unintended consequences.
Marc Zimmer, Professor of Chemistry, Connecticut College
This article is republished from The Conversation under a Creative Commons license. Read the original article.