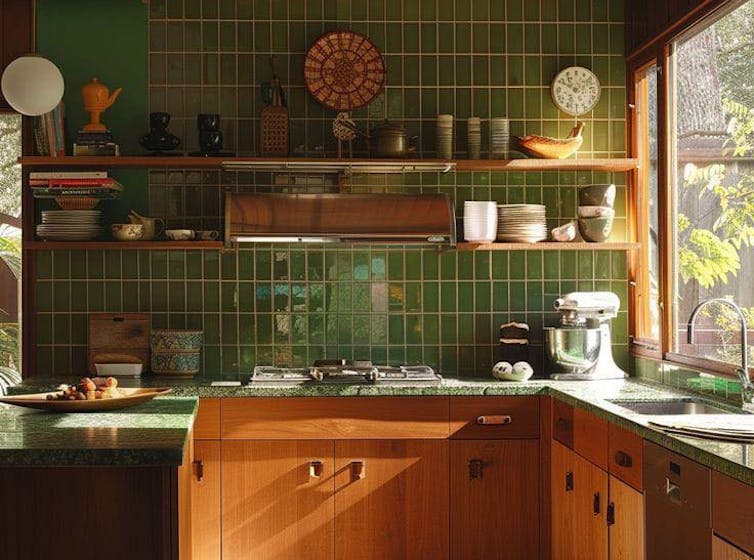
Tiny Homes via Facebook
Arryn Robbins, University of Richmond
I’m more of a scroller than a poster on social media. Like many people, I wind down at the end of the day with a scroll binge, taking in videos of Italian grandmothers making pasta or baby pygmy hippos frolicking.
For a while, my feed was filled with immaculately designed tiny homes, fueling my desire for minimalist paradise. Then, I started seeing AI-generated images; many contained obvious errors such as staircases to nowhere or sinks within sinks. Yet, commenters rarely pointed them out, instead admiring the aesthetic.
These images were clearly AI-generated and didn’t depict reality. Did people just not notice? Not care?
As a cognitive psychologist, I’d guess “yes” and “yes.” My expertise is in how people process and use visual information. I primarily investigate how people look for objects and information visually, from the mundane searches of daily life, such as trying to find a dropped earring, to more critical searches, like those conducted by radiologists or search-and-rescue teams.
With my understanding of how people process images and notice − or don’t notice − detail, it’s not surprising to me that people aren’t tuning in to the fact that many images are AI-generated.
We’ve been here before
The struggle to detect AI-generated images mirrors past detection challenges such as spotting photoshopped images or computer-generated images in movies.
But there’s a key difference: Photo editing and CGI require intentional design by artists, while AI images are generated by algorithms trained on datasets, often without human oversight. The lack of oversight can lead to imperfections or inconsistencies that can feel unnatural, such as the unrealistic physics or lack of consistency between frames that characterize what’s sometimes called “AI slop.”
Despite these differences, studies show people struggle to distinguish real images from synthetic ones, regardless of origin. Even when explicitly asked to identify images as real, synthetic or AI-generated, accuracy hovers near the level of chance, meaning people did only a little better than if they’d just guessed.
In everyday interactions, where you aren’t actively scrutinizing images, your ability to detect synthetic content might even be weaker.
Attention shapes what you see, what you miss
Spotting errors in AI images requires noticing small details, but the human visual system isn’t wired for that when you’re casually scrolling. Instead, while online, people take in the gist of what they’re viewing and can overlook subtle inconsistencies.
Visual attention operates like a zoom lens: You scan broadly to get an overview of your environment or phone screen, but fine details require focused effort. Human perceptual systems evolved to quickly assess environments for any threats to survival, with sensitivity to sudden changes − such as a quick-moving predator − sacrificing precision for speed of detection.
This speed-accuracy trade-off allows for rapid, efficient processing, which helped early humans survive in natural settings. But it’s a mismatch with modern tasks such as scrolling through devices, where small mistakes or unusual details in AI-generated images can easily go unnoticed.
People also miss things they aren’t actively paying attention to or looking for. Psychologists call this inattentional blindness: Focusing on one task causes you to overlook other details, even obvious ones. In the famous invisible gorilla study, participants asked to count basketball passes in a video failed to notice someone in a gorilla suit walking through the middle of the scene.
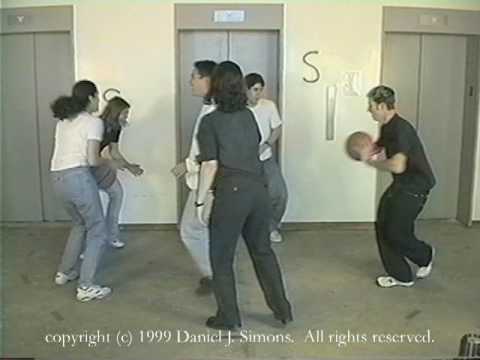
Similarly, when your focus is on the broader content of an AI image, such as a cozy tiny home, you’re less likely to notice subtle distortions. In a way, the sixth finger in an AI image is today’s invisible gorilla − hiding in plain sight because you’re not looking for it.
Efficiency over accuracy in thinking
Our cognitive limitations go beyond visual perception. Human thinking uses two types of processing: fast, intuitive thinking based on mental shortcuts, and slower, analytical thinking that requires effort. When scrolling, our fast system likely dominates, leading us to accept images at face value.
Adding to this issue is the tendency to seek information that confirms your beliefs or reject information that goes against them. This means AI-generated images are more likely to slip by you when they align with your expectations or worldviews. If an AI-generated image of a basketball player making an impossible shot jibes with a fan’s excitement, they might accept it, even if something feels exaggerated.
While not a big deal for tiny home aesthetics, these issues become concerning when AI-generated images may be used to influence public opinion. For example, research shows that people tend to assume images are relevant to accompanying text. Even when the images provide no actual evidence, they make people more likely to accept the text’s claims as true.
Misleading real or generated images can make false claims seem more believable and even cause people to misremember real events. AI-generated images have the power to shape opinions and spread misinformation in ways that are difficult to counter.
Beating the machine
While AI gets better at detecting AI, humans need tools to do the same. Here’s how:
- Trust your gut. If something feels off, it probably is. Your brain expertly recognizes objects and faces, even under varying conditions. Perhaps you’ve experienced what psychologists call the uncanny valley and felt unease with certain humanoid faces. This experience shows people can detect anomalies, even when they can’t fully explain what’s wrong.
- Scan for clues. AI struggles with certain elements: hands, text, reflections, lighting inconsistencies and unnatural textures. If an image seems suspicious, take a closer look.
- Think critically. Sometimes, AI generates photorealistic images with impossible scenarios. If you see a political figure casually surprising baristas or a celebrity eating concrete, ask yourself: Does this make sense? If not, it’s probably fake.
- Check the source. Is the poster a real person? Reverse image search can help trace a picture’s origin. If the metadata is missing, it might be generated by AI.
AI-generated images are becoming harder to spot. During scrolling, the brain processes visuals quickly, not critically, making it easy to miss details that reveal a fake. As technology advances, slow down, look closer and think critically.
Arryn Robbins, Assistant Professor of Psychology, University of Richmond
This article is republished from The Conversation under a Creative Commons license. Read the original article.